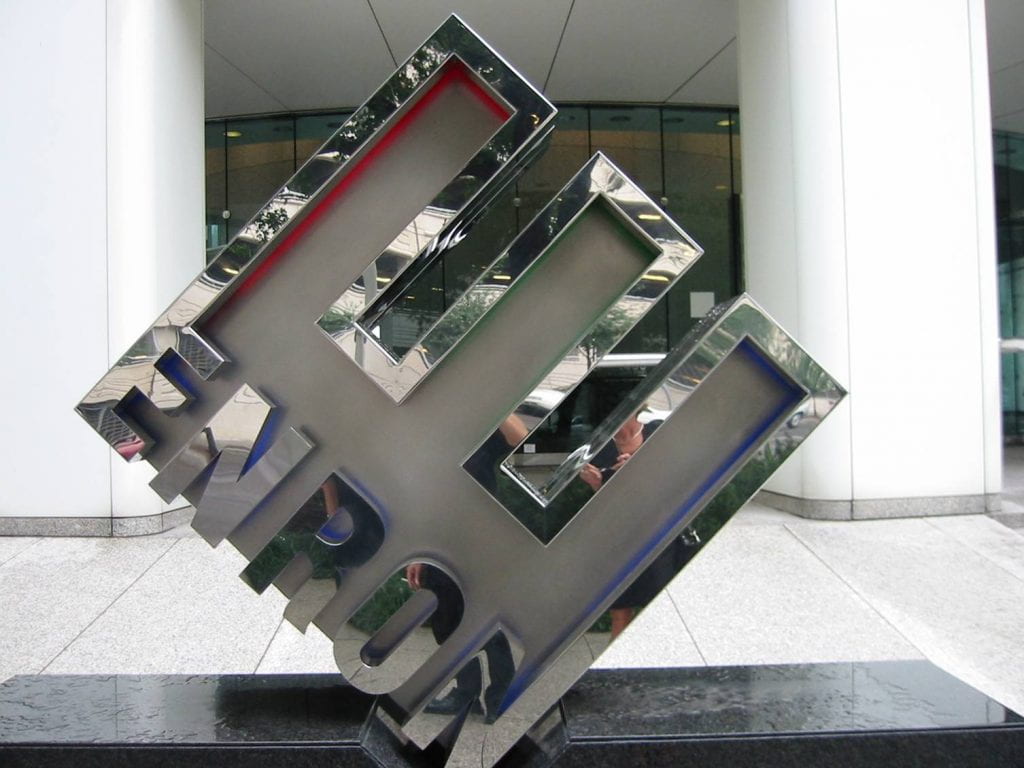
Beneish’s M-Score model was among the earliest to detect fraud at Enron. (Photo by Hanne Therkildsen)
BLOOMINGTON, Ind. — Enhanced oversight over the auditing profession and firms’ financial reporting has led to a proliferation of models to predict financial statement fraud.
But one of the first forensic models, the M-Score, devised by an Indiana University Kelley School of Business professor in the late 90s, remains accurate and is the most economically viable for investors to use, according to a forthcoming paper in The Accounting Review — the official journal of the American Accounting Association.
The article, “The Costs of Fraud Prediction Errors,” co-authored by M. Daniel Beneish, professor of accounting and the Alva L. Prickett Chair at Kelley, compares seven fraud prediction models with a cost-based measure that nets the benefits of correctly anticipating instances of fraud against the costs borne by incorrectly identifying non-fraud firms as fraudulent.
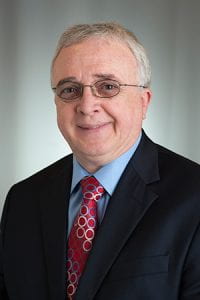
Daniel Beneish
Even though newer fraud models nearly doubled the success rate of M-Score, which Beneish developed, they did so at the cost of a much larger number of false positives. As a result, the other models are not used in practice by auditors because they are too costly to implement as all flagged firms must be carefully investigated.
“I have long known from my experience consulting with Arthur Andersen — for whom my model detected Enron before the debacle — and other public accounting firms, that litigation concerns relating to false positives — firms incorrectly flagged as having fraudulent financial statements — created an unwillingness by auditors’ general counsel to use fraud prediction models in practice,” Beneish said.
“My efforts back then to improve the M-Score in the context of auditing failed because I could not increase the model’s success rate without increasing the number of false positives. It seems that the new models cannot either,” he added.
Interestingly, as early as 2017 the M-Score flagged Kangmei Pharmaceutical, a Chinese publicly traded company that was involved in financial reporting fraud between 2016 and 2018. Like the Enron scandal in the U.S., the Kangmei Pharmaceutical scandal helped trigger new regulation in China that increased regulatory penalties for financial fraud (effective March 2020) and last November became China’s first successful class-action lawsuit involving corporate fraud. Its chairman was sentenced to 12 years in prison.
“The main purpose of our paper is to provide evidence on the costs and benefits of using fraud prediction models, and to show whether using these models is economically viable for auditors, investors and regulators,” Beneish said. “This is important because the traditional measures commonly used in recent research to justify new models are misleading about model performance in fraud samples as the proportion of fraud firms in the population is very small, and as they typically assume that the cost of a false positive and false negatives (missed detections) are equal.”
For example, assume that among 10,000 publicly traded firms, there are about 60 fraud firms and 9,940 firms without misreporting. The newer models detected 42 frauds (70% of the total frauds), and incorrectly flagged 3,976 firms (40% of the non-frauds). The latter is too large a number for most decision makers to investigate.
“Our evidence that a cost-based assessment of models is preferable to traditional model comparison measures (e.g., area under the curve), should become even more important as efforts by future researchers in the areas of data mining and machine learning intensify,” Beneish said.
Patrick Vorst of Maastricht University, assistant professor in financial accounting and accounting & information management, co-authored the paper with Beneish.
Daniel, toujours en avance
No me extrana que tu modelo sea todavia el mejor. So proud to be your sister, always!!